Tactical Insight Through Team Personas | An OptaPro Presentation
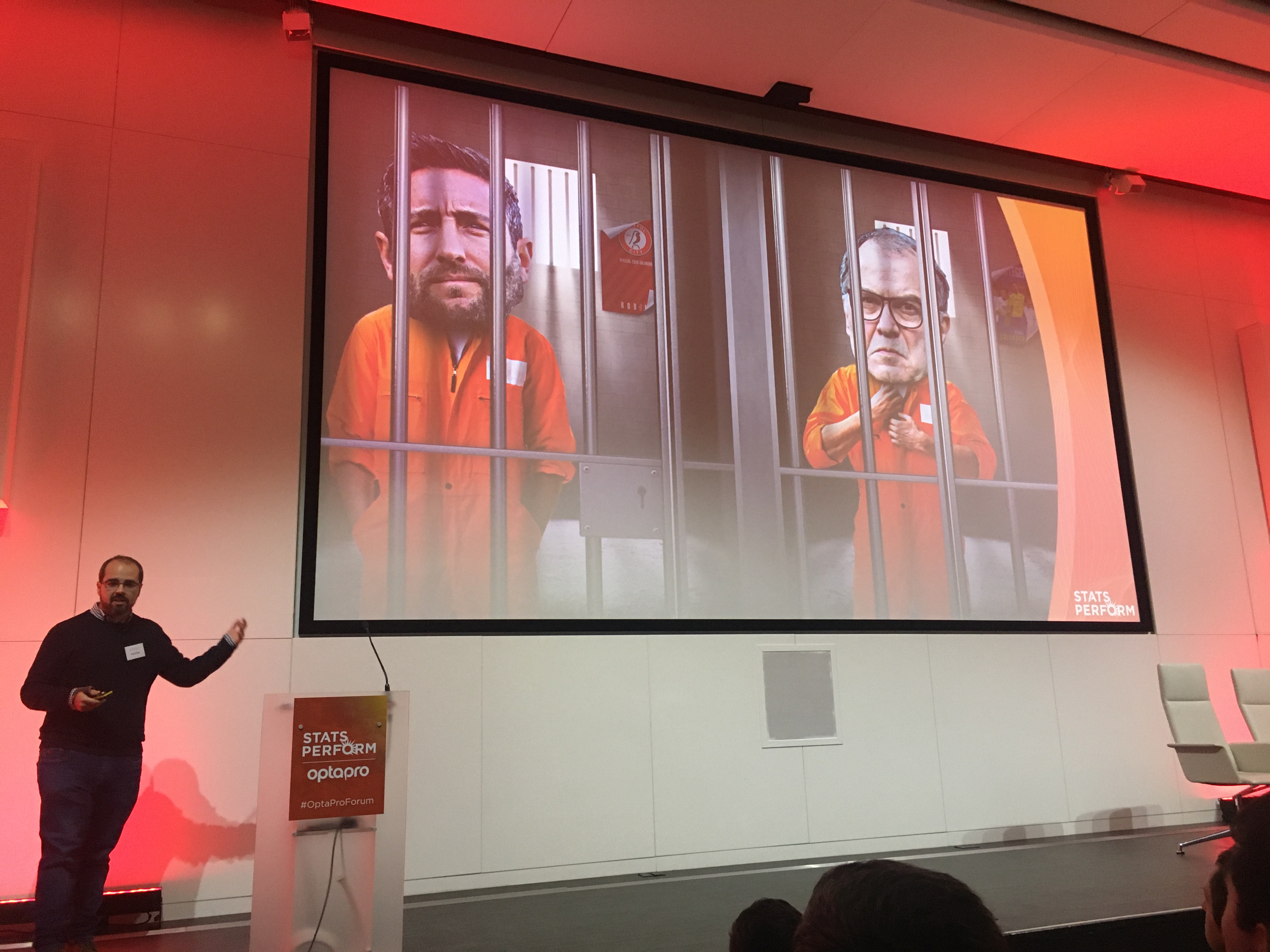
It’s one of the most important questions in football: if we expect our opposition to play in a certain way, what’s the most effective way for us to play against that? That’s what we at Twenty3 were trying to answer at this year’s Opta Pro Forum.
The Forum is an event held by data provider Opta, whose stats are used by Sky Sports, the Premier League, and more clubs and media organisations than Lionel Messi has match balls (though only just). It’s where the public get the chance to explore football’s big questions with data in front of the analysis staff of most clubs in England. We were mentored for this project ourselves by Tom Goodall from Swansea City, who’d specifically asked for submission proposals on this topic.
We tackled it in Blue Peter fashion using a statistical model we’d made earlier: David Perdomo Meza’s persona model. He’d unveiled personas at the Forum two years ago, but this project was using it for a specific analytical purpose.
The technique to create personas takes inspiration from natural language processing. It takes in the football data that Opta produces and identifies what, in language analysis, is called ‘topics’, but in football data analysis resembles styles of play. A team who make more long balls and aerial duels and fewer successful passes slot into the ‘long balls’ style, for example. Just like a passage of text won’t exclusively be about one topic, but will have features of several, so too does a football team have aspects of several styles, even if they have one that is dominant.
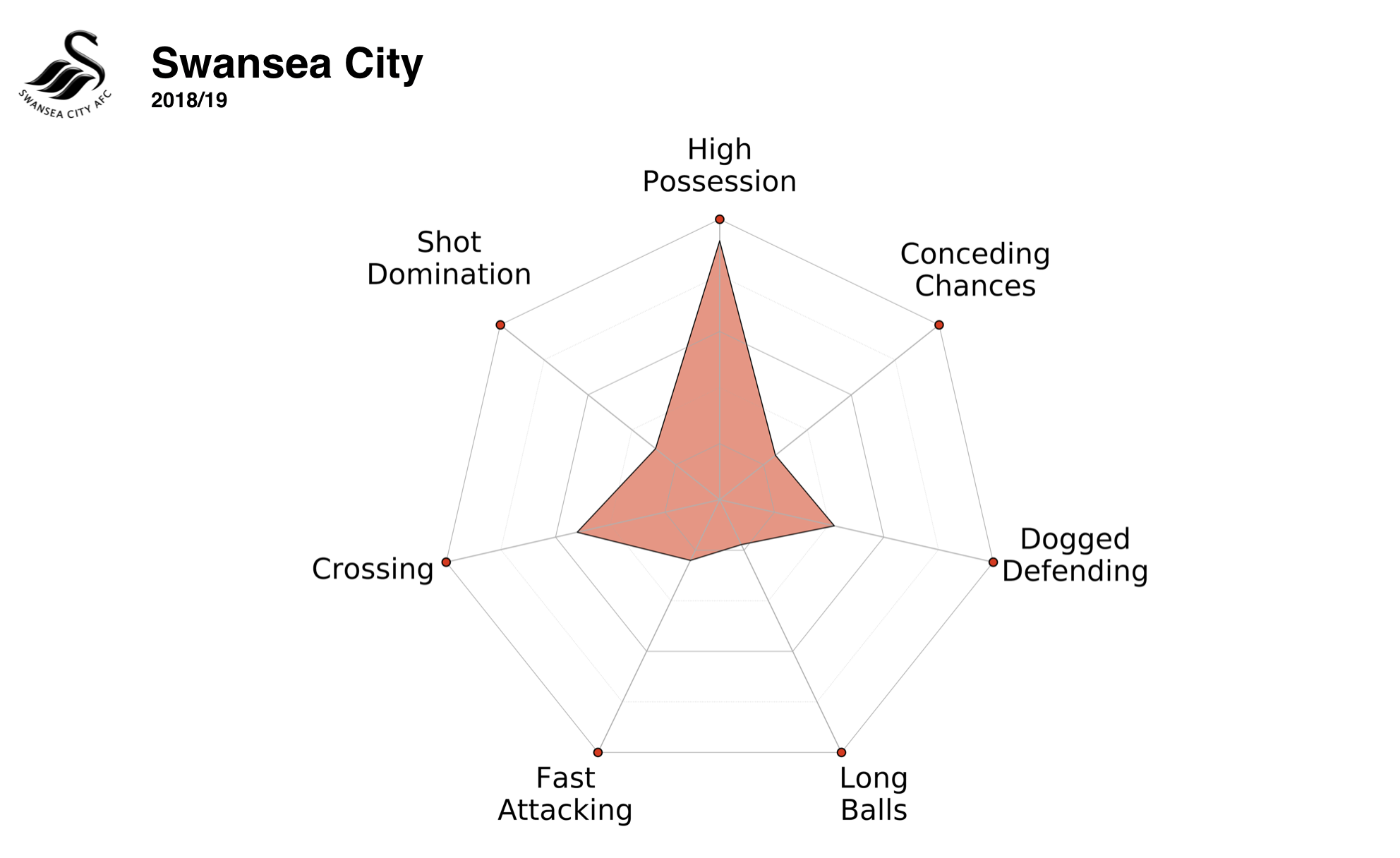
As a starting point, we calculated the personas for different formations, as played by teams in the Championship last season. Teams playing 4-4-2 are more of a long ball style than teams playing a 4-3-3 (surprise surprise), less of a possession style, but otherwise, things look pretty similar between them.
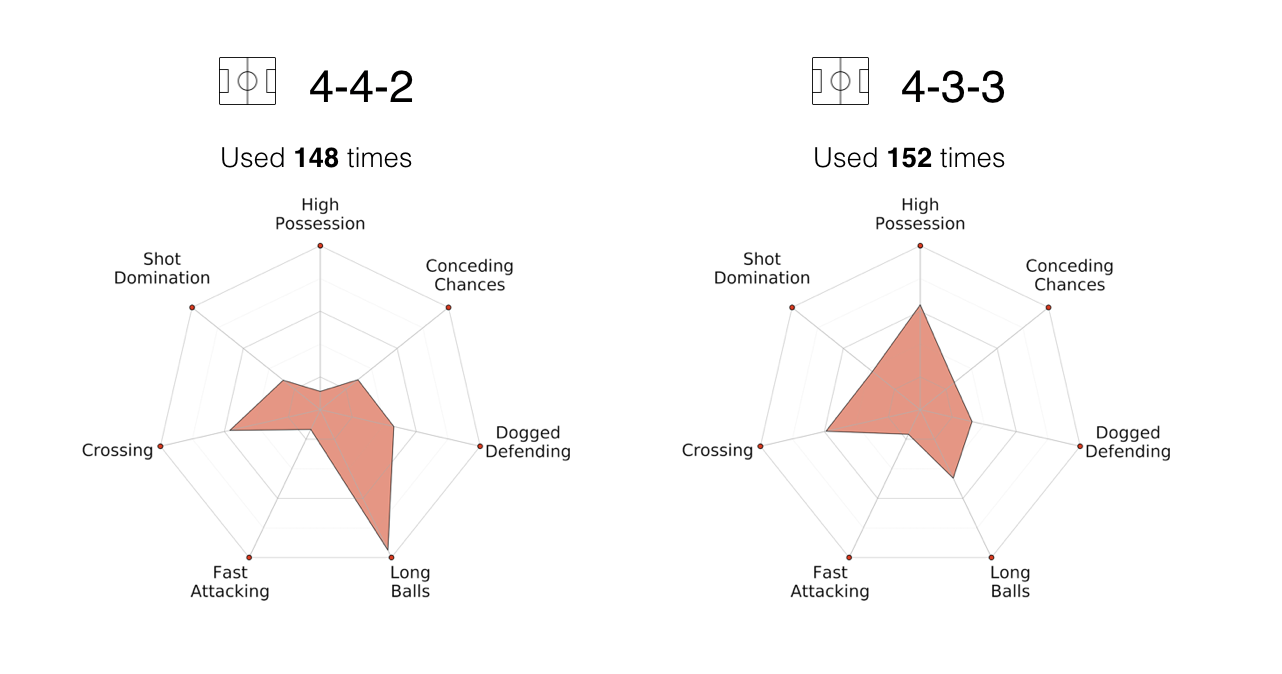
This is interesting, but we know that teams aren’t just about formations. Chris Wilder’s 3-5-2 with Sheffield United this season would be a different 3-5-2 to one that Pep Guardiola would play with Manchester City.
Instead of simply focusing on the nominal formation, we ran the personas through a clustering algorithm to get groups of styles. This meant that instead of one persona for every team in every match, each one pretty much unique, we had five groups that gathered similar personas together which we could compare easily.
The groups basically matched the five stylistic components of the persona model: High Possession, Dogged Defending, Long Balls, Fast Attacking, and Crossing (we’d taken Shot Domination and Conceding Chances out of the equation earlier on, so that we were solely focusing on style of play). Here’s an example of a match from the Crossing cluster from Swansea’s game against Bristol City earlier in the season — sure enough, the flank attacks visualisation shows that most of their attacking, in terms of frequency and threat, came down the wings.
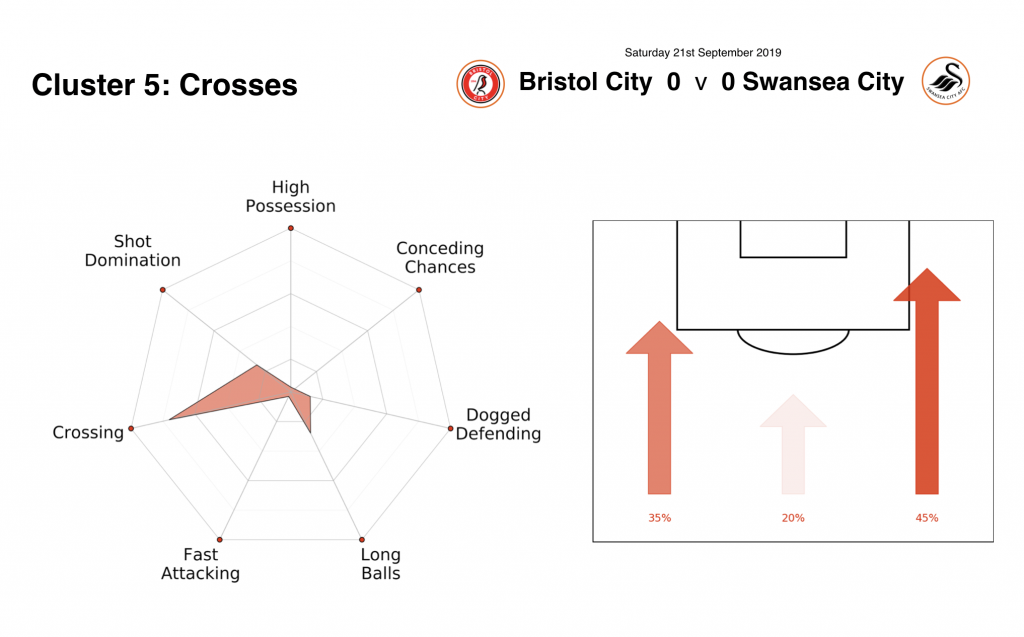
With our personas now in nice little groups, we could look at how the groups matched up against each other. Importantly, before we did this we created a model to take team strength out of the equation — no use saying that possession is the way to go if your model is only noticing that Liverpool and Manchester City are the sides who make the most passes. All of these examples and trade-offs below, then, are purely about the tactical style.
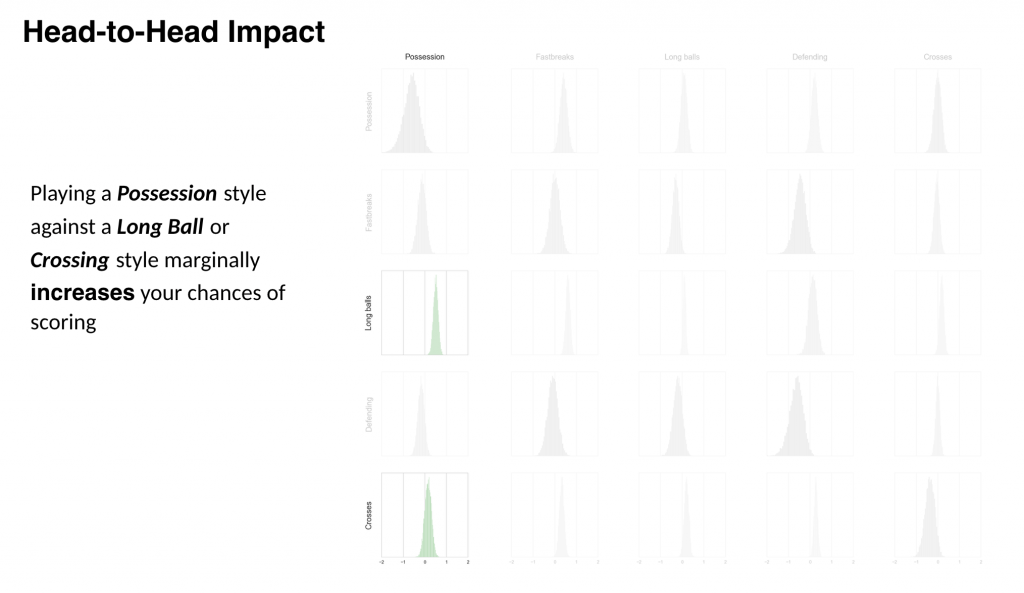
The above only shows how this stylistic match-up affects chances of scoring, but it’s interesting to note that sometimes teams care more about stopping their opponents from scoring than scoring themselves. If you’re facing a possession-based team, then our persona analysis found that playing in a possession style yourself was the best way to reduce their chances of scoring.
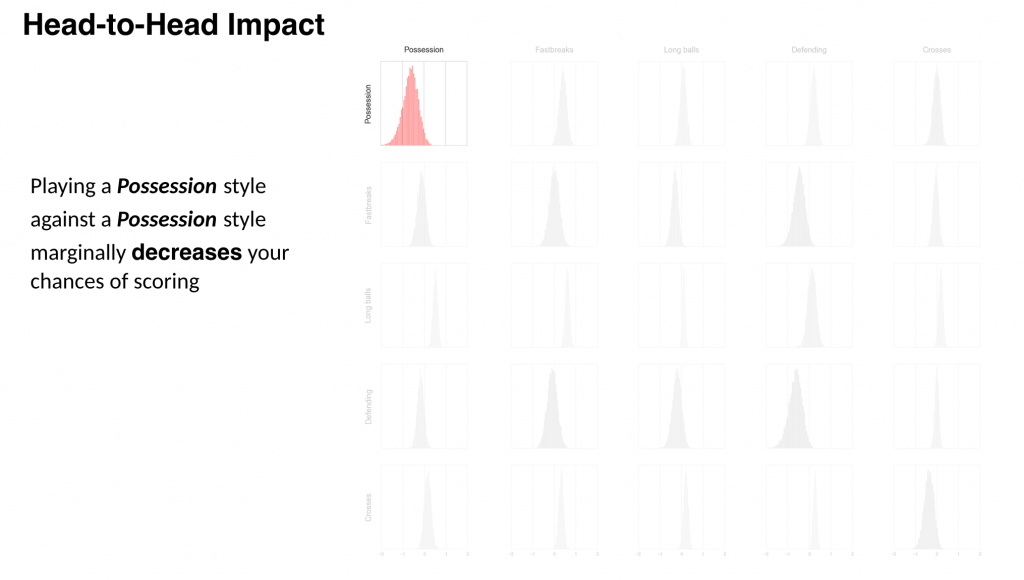
Sure, it also reduces your own chance of adding to the score, but if you’re happy to sit on a lead, this looks to be the way to go.
Putting this all together — attack and defence, yourselves and your opponents — can throw up some interesting conundrums. There are match-ups where either team has two main styles, and these two styles have different match-up values. Team 1’s main style may be better against Team 2’s main style, but Team 1’s back-up is the better choice if Team 2 plays their back-up.
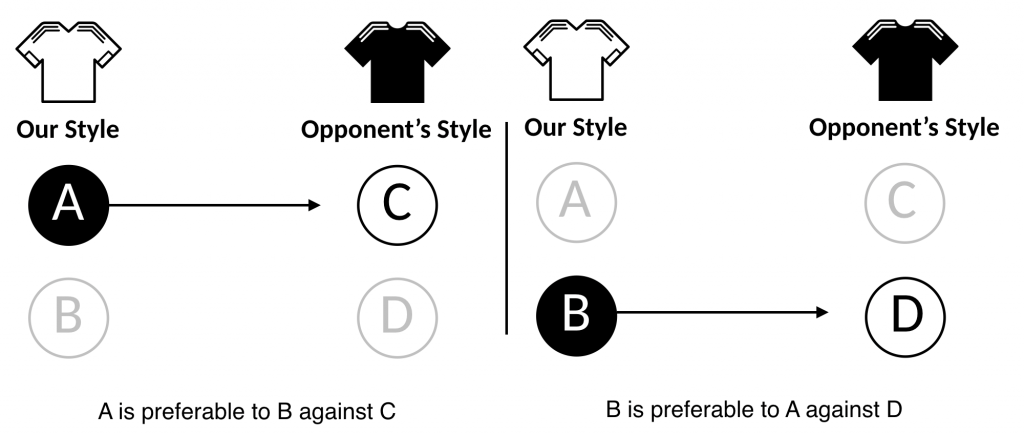
There are various ways that teams can tell what style their opponent is likely to play, based on our models. Teams may be more prone to playing in a particular group in one formation, while they play another style in a different formation. There might also be players who are linked to a stylistic group, and when that individual is on the pitch their team is much more likely to play in that style than when they’re off it.
Ultimately, the big thing that comes out of this project is a model and a framework that teams could use to work out what style to play in a particular match. It’s not going to be something that’ll make world-beaters out of a League Two team, but the insight that the personas can add could be enough to move the needle of probabilities a few percentage points in your favour. In professional sport, every little movement of the needle counts.